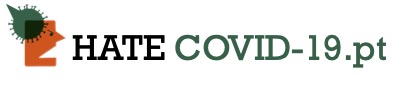
Most approaches on hate speech detection focus on direct hatred content, neglecting indirect or veiled hate speech. Moreover, automated methods usually rely on annotated corpora, which are still scarce in Portuguese and useless to study spatiotemporally delimited issues, such as the Covid-19 pandemic. This project seeks to contribute to the analysis and detection of online hate speech in Portuguese, investigating the linguistic and rhetorical strategies underlying both overt and covert hatred content. Specifically, we will create a large annotated corpus from social media, covering the Covid-19 pandemic, which will support the development of a machine learning prototype to detect hate speech, and assess its explicitness and intensity, considering the time period and geolocation data.
Goals
The key objectives of this project are twofold. Firstly, it attempts to develop methods for semi-automatically creating a large-scale Portuguese annotated corpus covering both overt and covert online hate speech, before and during the Covid-19 pandemic. Secondly, it intends to create a prototype that demonstrates how the information in the annotated corpus can support hate speech detection, allowing users to visualize the metrics extracted from data, considering attributes like hate speech target and publication date, and highlighting the linguistic and rhetorical clues underlying hatred content. The developed resources and tools will promote the research on the dynamics of online hate speech in Portuguese, particularly in adverse contexts, such as a pandemic outbreak.
Description
Most research in hate speech detection focuses on the creation of linguistic resources, particularly annotated corpora, and on the development of methods and tools for automatically detecting offensive or abusive language [1].
However, the lack of consensus on the definition and characterization of hate speech has led to the creation of heterogeneous resources and approaches, based on different semantic classification systems, making it difficult to compare them [2]. In addition, hate speech is intrinsically dependent on the sociocultural context, which means that existing resources cannot be directly transferable or easily adaptable to other linguistic and pragmatic contexts. Although there are few corpora specifically designed for detecting hate speech in Portuguese [3], their usefulness is quite limited if we want to study spatiotemporally delimited phenomena, such as the expression of hate by the Portuguese online community during the Covid-19 pandemic.
Moreover, existing corpora are often created based on elementary lexical-based approaches, using closed lists of keywords with negative polarity, typically involving epithets and slurs to incite hatred or violence against a group. This selection method leaves out an immense set of potentially relevant hatred content, preventing an in-depth understanding of the nature and extent of this phenomenon. Furthermore, most research focuses on the analysis and detection of direct and overt hate speech, neglecting other productive forms of hate speech, such as indirect and covert hate speech. The latter usually uses irony, analogy and humor, posing additional challenges to human and automated recognition.
To address the research gaps mentioned above, we will create a representative corpus covering online hate speech before and during Covid-19 pandemic in Portugal. This corpus will be finely-grained annotated, considering several dimensions related to hate speech. The annotation will be performed by trained annotators, following specific guidelines that will be created by the project’s team and then validated by experts from social sciences. In addition to manual annotation, which will be subject to a thorough inter-annotator agreement study, we will apply transfer-learning methods to automatically enlarge the annotated corpus [4]. The annotations from corpora will support the development of machine learning classifiers capable of identifying and monitoring both overt and veiled hate speech in Portuguese social media.